The human brain isn’t magic; nor are the problem-solving abilities our brains possess. They are, however, still poorly understood. If there’s nothing magical about our brains or essential about the carbon atoms that make them up, then we can imagine eventually building machines that possess all the same cognitive abilities we do. Despite the recent advances in the field of artificial intelligence, it is still unclear how we might achieve this feat, how many pieces of the puzzle are still missing, and what the consequences might be when we do. There are, I will argue, good reasons to be concerned about AI.
The Capabilities Challenge
While we lack a robust and general theory of intelligence of the kind that would tell us how to build intelligence from scratch, we aren’t completely in the dark. We can still make some predictions, especially if we focus on the consequences of capabilities instead of their construction. If we define intelligence as the general ability to figure out solutions to a variety of problems or identify good policies for achieving a variety of goals, then we can reason about the impacts that more intelligent systems could have, without relying too much on the implementation details of those systems.
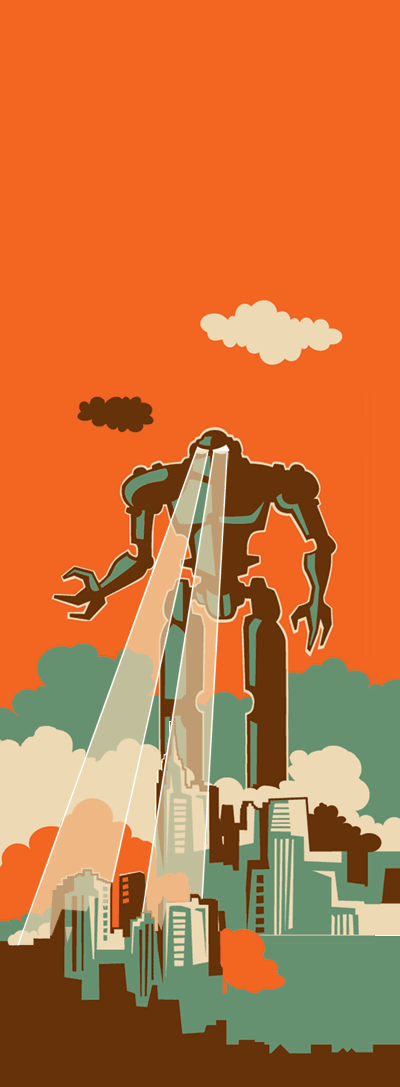
Our intelligence is ultimately a mechanistic process that happens in the brain, but there is no reason to assume that human intelligence is the only possible form of intelligence. And while the brain is complex, this is partly an artifact of the blind, incremental progress that shaped it—natural selection. This suggests that developing machine intelligence may turn out to be a simpler task than reverse- engineering the entire brain. The brain sets an upper bound on the difficulty of building machine intelligence; work to date in the field of artificial intelligence sets a lower bound; and within that range, it’s highly uncertain exactly how difficult the problem is. We could be 15 years away from the conceptual breakthroughs required, or 50 years away, or more.
The fact that artificial intelligence may be very different from human intelligence also suggests that we should be very careful about anthropomorphizing AI. Depending on the design choices AI scientists make, future AI systems may not share our goals or motivations; they may have very different concepts and intuitions; or terms like “goal” and “intuition” may not even be particularly applicable to the way AI systems think and act. AI systems may also have blind spots regarding questions that strike us as obvious. AI systems might also end up far more intelligent than any human.
The last possibility deserves special attention, since superintelligent AI has far more practical significance than other kinds of AI.
AI researchers generally agree that superintelligent AI is possible, though they have different views on how and when it’s likely to be developed. In a 2013 survey, top-cited experts in artificial intelligence assigned a median 50% probability to AI being able to “carry out most human professions at least as well as a typical human” by the year 2050, and also assigned a 50% probability to AI greatly surpassing the performance of every human in most professions within 30 years of reaching that threshold.
Many different lines of evidence and argument all point in this direction; I’ll briefly mention just one here, dealing with the brain’s status as an evolved artifact. Human intelligence has been optimized to deal with specific constraints, like passing the head through the birth canal and calorie conservation, whereas artificial intelligence will operate under different constraints that are likely to allow for much larger and faster minds. A digital brain can be many orders of magnitude larger than a human brain, and can be run many orders of magnitude faster.
All else being equal, we should expect these differences to enable (much) greater problem-solving ability by machines. Simply improving on human working memory all on its own could enable some amazing feats. Examples like arithmetic and the game Go confirm that machines can reach superhuman levels of competency in narrower domains, and that this competence level often follows swiftly after human-par performance is achieved.
The Alignment Challenge
If and when we do develop general-purpose AI, or artificial general intelligence (AGI), what are the likely implications for society? Human intelligence is ultimately responsible for human innovation in all walks of life. The prospect of developing machines that can dramatically accelerate our rate of scientific and technological progress is a prospect of incredible growth from this engine of prosperity.
Our ability to reap these gains, however, depends on our ability to design AGI systems that are not only good at solving problems, but oriented toward the right set of problems. A highly capable, highly general problem-solving machine would function like an agent in its own right, autonomously pursuing whatever goals (or answering whatever questions, proposing whatever plans, etc.) are represented in its design. If we build our machines with subtly incorrect goals (or questions, or problem statements), then the same general problem-solving ability that makes AGI a uniquely valuable ally may make it a uniquely risky adversary.
Why an adversary? I’m not assuming that AI systems will resemble humans in their motivations or thought processes. They won’t necessarily be sentient (unless this turns out to be required for high intelligence), and they probably won’t share human motivations like aggression or a lust for power.
There do, however, seem to be a number of economic incentives pushing toward the development of ever-more-capable AI systems granted ever-greater autonomy to pursue their assigned objectives. The better the system is at decisionmaking, the more one gains from removing humans from the loop, and the larger the push towards autonomy. (See, for example, this article on why tool AIs want to be agent AIs.) There are also many systems in which having no human in the loop leads to better standardization and lower risk of corruption, such as assigning a limited supply of organs to patients. As our systems become smarter, human oversight is likely to become more difficult and costly; past a certain level, it may not even be possible, as the complexity of the policies or inventions an AGI system devises surpasses our ability to analyze their likely consequences.
AI systems are likely to lack human motivations such as aggression, but they are also likely to lack the human motivations of empathy, fairness, and respect. Their decision criteria will simply be whatever goals we design them to have; and if we misspecify these goals even in small ways, then it is likely that the resultant goals will not only diverge from our own, but actively conflict with them.
The basic reason to expect conflict (assuming we fail to perfectly specify our goals) is that it appears to be a technically difficult problem to specify goals that aren’t open-ended and ambitious; and sufficiently capable pursuit of sufficiently open-ended goals implies that strategies such as “acquire as many resources as possible” will be highly ranked by whatever criteria the machine uses to make decisions.
Why do ambitious goals imply “greedy” resource acquisition? Because physical and computational resources are broadly helpful for getting things done, and are limited in supply. This tension naturally puts different agents with ambitious goals in conflict, as human history attests—except in cases where the agents in question value each other’s welfare enough to wish to help one another, or are at similar enough capability levels to benefit more from trade than from resorting to force. AI raises the prospect that we may build systems with “alien” motivations that don’t overlap with any human goal, while superintelligence raises the prospect of unprecedentedly large capability differences.
Even a simple question-answering system poses more or less the same risks on those fronts as an autonomous agent in the world, if the question-answering system is “ambitious” in the relevant way. It’s one thing to say (in English) “we want you to answer this question about a proposed power plant design in a reasonable, common-sense way, and not build in any covert subsystems that would make the power plant dangerous;” it’s quite another thing to actually specify this goal in code, or to hand-code patches for the thousand other loopholes a sufficiently capable AI system might find in the task we’ve specified for it.
If we build a system to “just answer questions,” we need to find some way to specify a very non-ambitious version of that goal. If not we risk building a system with incentives to seize control and maximize the number of questions it receives, maximize the approval ratings it receives from users, or otherwise to maximize some quantity that correlates with good performance in training data and is likely to come uncorrelated in the real world.
Why, then, does it look difficult to specify non-ambitious goals? Because our standard mathematical framework of decision-making—expected utility maximization—is built around ambitious, open-ended goals. When we try to model a limited goal (for example, “just put a single strawberry on a plate and then stop there, without having a big impact on the world,”) expected utility maximization is a poor fit. It’s always possible to keep driving up the expected utility higher and higher by devising evermore- ingenious ways to increment the probability of your success; and if your machine is smarter than you are, and all it cares about is this success criterion you’ve given it, then “crazy”-sounding ideas like “seize the world’s computing resources and run millions of simulations of possible ways I might be wrong about whether the strawberry is on the plate, just in case,” will be highly ranked by this supposedly “unambitious” goal.
Researchers are considering a number of different ideas for addressing this problem, and we’ve seen some progress over the last couple of years, but it’s still largely an unsolved and under-studied problem. We could consider adding a penalty term to any policies the system comes up with that have a big impact on the world—but defining “impact” in a useful way turns out to be a very difficult problem.
One could try to design systems to only “mildly” pursue their goals, such as stopping the search for ever-better policies once a policy that hits a certain expected utility threshold is found. But systems of this kind, called “satisficers,” turn out to run into some difficult obstacles of their own. Most obviously, naïve attempts at building a satisficer may give the system incentives to write and run the code for a highly capable non-satisficing sub-agent, since a maximizing sub-agent can be a highly effective way to satisfice for a goal.
For a summary of these and other technical obstacles to building superintelligent but “unambitious” machines, see Taylor et al.’s article “Alignment for Advanced Machine Learning Systems”.
Alignment Through Value Learning
Why can’t we just build ambitious machines that share our values?
Ambition in itself is no vice. If we can successfully instill everything we want into the system, then there’s no need to fear open-ended maximization behavior, because the scary edge-case scenarios we’re worried about will be things the AI system itself knows to worry about too. Similarly, we won’t need to worry about an aligned AI with sufficient foresight modifying itself to be unaligned, or creating unaligned descendents because it will realize that doing so would go against its values.
The difficulty is that human goals are complex, varied, and situation-dependent. Coding them all by hand is a non-starter. (And no, Asimov’s three laws of robotics are not a plausible design proposal for real-world AI systems. Many of the books explored how they didn’t work, and in any case they were there mainly as plot devices!)
What we need, then, would seem to be some formal specification of a process for learning human values over time. This task has itself raised a number of surprisingly deep technical challenges for AI researchers.
Many modern AI systems, for example, are trained using reinforcement learning. A reinforcement learning system builds a model of how the world works through exploration and feedback rewards, trying to collect as much reward as it can. One might think that we could just keep using these systems as capabilities ratchet past the human level, rewarding AGI systems for behaviors we like and punishing them for behaviors we dislike, much like raising a human child.
This plan runs into several crippling problems, however. I’ll discuss two: defining the right reward channel, and ambiguous training data.
The end goal that we actually want to encourage through value learning is that the trainee wants the trainer to be satisfied, and we hope to teach this by linking the trainer’s satisfaction with some reward signal. For dog training, this is giving a treat; for a reinforcement learning system, it might be pressing a reward button. The reinforcement learner, however, has not actually been designed to satisfy the trainer, or to promote what the trainer really wants. Instead, it has simply been built to optimize how often it receives a reward. At low capability levels, this is best done by cooperating with the trainer; but at higher capability levels, if it could use force to seize control of the button and give itself rewards, then solutions of this form would be rated much more highly than cooperative solutions. To have traditional methods in AI safely scale up with capabilities, we need to somehow formally specify the difference between the trainer’s satisfaction and the button being pressed, so that the system will see stealing the button and pressing it directly as irrelevant to its real goal. This is another example of an open research question; we don’t know how to do this yet, even in principle.
We want the system to have general rules that hold across many contexts. In practice, however, we can only give and receive specific examples in narrow contexts. Imagine training a system that learns how to classify photos of everyday objects and animals; when presented with a photo of a cat, it confidently asserts that the photo is of a cat. But what happens when you show it a cartoon drawing of a cat? Whether or not the cartoon is a “cat” depends on the definition that we’re using—it is a cat in some senses, but not in others. Since both concepts of “cat” agree that a photo of a cat qualifies, just looking at photos of cats won’t help the system learn what rule we really have in mind. In order for us to predict all the ways that training data might under-specify the rules we have in mind, however, it would seem that we’d need to have superhuman foresight about all the complex edge cases that might ever arise in the future during a real-world system’s deployment.
While it seems likely that some sort of childhood or apprenticeship process will be necessary, our experience with humans, who were honed by evolution to cooperate in human tribes, is liable to make us underestimate the practical difficulty of rearing a non-human intelligence. And trying to build a “human-like” AI system, without first fully understanding what makes humans tick could make the problem worse. The system may still be quite inhuman under the hood, while its superficial resemblance to human behavior further encourages our tendency to anthropomorphize the system and assume it will always behave in human-like ways.
For more details on these research directions within AI, curious readers can check out Amodei, et al.’s “Concrete Problems in AI Safety”, along with the Taylor et al. paper above.
The Big Picture
At this point, I’ve laid out my case for why I think superintelligent AGI is likely to be developed in the coming decades, and I’ve discussed some early technical research directions that seem important for using it well. The prospect of researchers today being able to do work that improves the long-term reliability of AI systems is a key practical reason why AI risk is an important topic of discussion today. The goal is not to wring our hands about hypothetical hazards, but to calmly assess their probability (if only heuristically) and actually work to resolve the hazards that seem sufficiently likely.
A reasonable question at this point is whether the heuristics and argument styles I’ve used above to try and predict a notional technology, general-purpose AI, are likely to be effective. One might worry, for example—as Michael Shermer does in this issue of Skeptic—that the scenario I’ve described above, however superficially plausible, is ultimately a conjunction of a number of independent claims.
A basic tenet of probability theory is that conjunctions are necessarily no more likely than their individual parts; the claim “Linda is a feminist bank teller” cannot be more likely than the claim “Linda is a feminist” or the claim “Linda is a bank teller,” in this now famous cognitive bias experiment by Amos Tversky and Daniel Kahneman. This is true here as well; if any of the links above are wrong, the entire chain fails.
A quirk of human psychology is that corroborative details can often make a story feel as though it is likelier, by making it more vivid and easier to visualize. If I suppose that the U.S. and Russia might break off diplomatic relations in the next five years, this might seem low probability; if I suppose that over the next five years the U.S. might shoot down a Russian plane over Syria and then that will lead to the countries breaking off diplomatic relations, this story might seem more likely than the previous one, because it has an explicit causal link. And indeed, studies show that people will generally assign a higher probability to the latter claim if two groups are randomly assigned one or the other claim in isolation. Yet the latter story is necessarily less likely—or at least no more likely—because it now contains an additional (potentially wrong) fact.
I’ve been careful in my argument so far to make claims not about pathways, which paint a misleadingly detailed picture, but about destinations. Destinations are disjunctive, in that many independent paths can all lead there, and so are as likely as the union of all the constituent probabilities. Artificial general intelligence might be reached because we come up with better algorithms on blackboards, or because we have continuing hardware growth, or because neuroimaging advances allow us to better copy and modify various complicated operations in human brains, or by a number of other paths. If one of those pathways turns out to be impossible or impractical, this doesn’t mean we can’t reach the destination, though it may affect our timelines and the exact capabilities and alignment prospects of the system. Where I’ve mentioned pathways, it’s been to help articulate why I think the relevant destinations are reachable, but the outlined paths aren’t essential.
This also applies to alignment. Regardless of the particular purposes we put AI systems to, if they strongly surpass human intelligence, we’re likely to run into many of the same difficulties with ensuring that they’re learning the right goals, as opposed to learning a close approximation of our goal that will eventually diverge from what we want. And for any number of misspecified goals highly capable AI systems might end up with, resource constraints are likely to create an adversarial relationship between the system and its operators.
To avoid inadvertently building a powerful adversary, and to leverage the many potential benefits of AI for the common good, we will need to find some way to constrain AGI to pursue limited goals or to employ limited resources; or we will need to find extremely reliable ways to instill AGI systems with our goals. In practice, we will surely need both, along with a number of other techniques and hacks for driving down risk to acceptable levels.
Why Work On This Now?
Suppose that I’ve convinced you that AGI alignment is a difficult and important problem. Why work on it now?
One reason is uncertainty. We don’t know whether it will take a short or long time to invent AGI, so we should prepare for short horizons as well as long ones. And just as we don’t know what work is left to do in order to make AGI, we don’t know what work is left to do in order to align AGI. This alignment problem, as it is called, may turn out to be more difficult than expected, and the sooner we start, the more slack we have. And if it proves unexpectedly easy, that means we can race ahead faster on capability development once we’re confident we can use them well.
On the other hand, starting work early means that we know less about what AGI will look like, and our safety work is correspondingly less informed. The research problems outlined above, however, seem fairly general: they’re likely to be applicable to a wide variety of possible designs. Once we have exhausted the low-hanging fruit and run out of obvious problems to tackle, the cost-benefit comparison here may shift.
Another reason to prioritize early alignment work is that AI safety may help shape capabilities research in critical respects.
One way to think about this is technical debt, a programming term used to refer to the development work that becomes necessary later because a cheap and easy approach was used instead of the right approach. One might imagine a trajectory where we increase AI capabilities as rapidly as possible, reach some threshold capability level where there is a discontinuous increase in the dangers (e.g., strong self-improvement capabilities), and then halt all AI development, focusing entirely on ensuring that the system in question is aligned before continuing development. This approach, however, runs into the same challenges as designing a system first for functionality, and then later going back and trying to “add in” security. Systems that aren’t built for high security at the outset generally can’t be made highly secure (at reasonable cost and effort) by “tacking on” security features much later on.
As an example, we can consider how strings were implemented in the C language, a general-purpose, imperative computer programming language. Developers chose the easier, cheaper way instead of the more secure way, leading to countless buffer overflow vulnerabilities that were painful to patch in systems that used C. Figuring out the sort of architecture a system needs to have and then building using that architecture seems to be much more reliable than building an architecture and hoping that it can be easily modified to also serve another purpose. We might find that the only way to build an alignable AI is to start over with a radically different architecture.
Consider three fields that can be thought of as normal fields under conditions of unusual stress:
- Computer security is like computer programming and mathematics, except that it also has to deal with the stresses imposed by intelligent adversaries. Adversaries can zero in on weaknesses that would only come up occasionally by chance, making ordinary “default” levels of exploitability highly costly in security-critical contexts. This is a major reason why computer security is famously difficult: you don’t just have to be clear enough for the compiler to understand; you have to be airtight.
- Rocket science is like materials science, chemistry, and mechanical engineering, except that it requires correct operation under immense pressures and temperatures on short timescales. Again, this means that small defects can cause catastrophic problems, as tremendous amounts of energy that are supposed to be carefully channeled end up misdirected.
- Space probes that we send on exploratory missions are like regular satellites, except that their distance from Earth and velocity put them permanently out of reach. In the case of satellites, we can sometimes physically access the system and make repairs. This is more difficult for distant space probes, and is often impossible in practice. If we discover a software bug, we can send a patch to a probe—but only if the antenna is still receiving signals, and the software that accepts and applies patches is still working. If not, your system is now an inert brick hurtling away from the Earth.
Loosely speaking, the reason AGI alignment looks difficult is that it shares core features with the above three disciplines.
- Because AGI will be applying intelligence to solve problems, it will also be applying intelligence to find shortcuts to the solution. Sometimes the shortcut helps the system find unexpectedly good solutions; sometimes it helps the system find unexpectedly bad ones, as when our intended goal was imperfectly specified. As with computer security, the difficulty we run into is that our goals and safety measures need to be robust to adversarial behavior. We can in principle build non-adversarial systems (e.g., through value learning or by formalizing limited-scope goals), and this should be the goal of AI researchers; but there’s no such thing as perfect code, and any flaw in our code opens up the risk of creating an adversary.
- More generally speaking, because AGI has the potential to be much smarter than people and systems that we’re used to and to discover technological solutions that are far beyond our current capabilities, safety measures we create for subhuman or human-par AI systems are likely to break down as these capabilities dramatically increase the “pressure” and “temperature” the system has to endure. For practical purposes, there are important qualitative differences between a system that’s smart enough to write decent code, and one that isn’t; between one that’s smart enough to model its operators’ intentions, and one that isn’t; between one that isn’t a competent biochemist, and one that is. This means that the nature of progress in AI makes it very difficult to get safety guarantees that scale up from weaker systems to smarter ones. Just as safety measures for aircraft may not scale to spacecraft, safety measures for low-capability AI systems operating in narrow domains are unlikely to scale to general AI.
- Finally, because we’re developing machines that are much smarter than we are, we can’t rely on after-the-fact patches or shutdown buttons to ensure good outcomes. Loss-of-control scenarios can be catastrophic and unrecoverable. Minimally, to effectively suspend a superintelligent system and make repairs, the research community first has to solve a succession of open problems. We need a stronger technical understanding of how to design systems that are docile enough to accept patches and shutdown operations, or that have carefully restricted ambitions or capabilities. Work needs to begin early exactly because so much of the work operates as a prerequisite for safely making further safety improvements to highly capable AI systems.
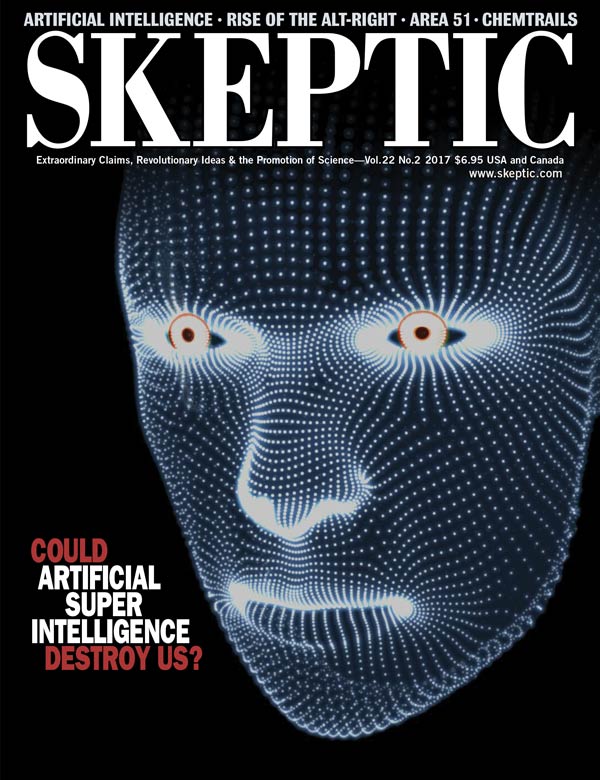
This article appeared in Skeptic magazine 22.2 (2017)
Buy print issue
Buy digital issue
Subscribe to print edition
Subscribe to digital edition
This looks like a hard problem. The problem of building AGI in the first place, of course, also looks hard. We don’t know nearly enough about either problem to say which is more difficult, or exactly how work on one might help inform work on the other. There is currently far more work going into advancing capabilities than advancing safety and alignment, however; and the costs of underestimating the alignment challenge far exceed the costs of underestimating the capabilities challenge. For that reason, this should probably be a more mainstream priority, particularly for AI researchers who think that the field has a very real chance of succeeding in its goal of developing general and adaptive machine intelligence.
About the Author
Matthew Graves is a staff writer at the Machine Intelligence Research Institute in Berkeley, CA. Previously, he worked as a data scientist, using machine learning techniques to solve industrial problems. He holds a master’s degree in Operations Research from the University of Texas at Austin.
This article was published on November 8, 2017.
Most of AI today is little more than elaborate look up tables. It’s barely computing.
>AI systems are likely to lack human motivations such as aggression, but they are also likely to lack the human motivations of empathy, fairness, and respect.
A system w/o emotions will not be intelligent and how to ‘simulate’ them is a much harder problem than merely solving problems.
Automation is a more urgent problem than actual intelligence. There are already ‘dark’ factories and self-driving uber cars. In airliners the pilots are just there to comfort the passengers.
Why don’t people strive to acquire all power and resources availabe? Not because of enumerate limitations and governors.
Because we recognize there are competing outcomes both within ourselves and externally.
When it comes to the pursuit of the technology, they knew theoretically E=mc^2 and still made the bomb. How much more likely to advance AI into the unknown driven by utopia and restricted by paranoia. Neither of which is good reason for making any decision.
Like burning fossil fuels we had no real world idea where it would lead us. So, it will be with AI and when we get to the end we will not have arrived.
Awc
I still remain skeptical about the abilities of computers, however data-saturated they may get. Suppose we entered the information from everything that has ever been published or publicly articulated, from all the libraries and databases and websites in the world, into a single computer, and programed it with the ability to cross-reference all of that information — something which would be far, far, far beyond the capacity of any human being. Would that data-saturated computer have “intelligence” in any practical sense, much less “understanding” of all that data, much less “consciousness,” much less “desires” or “motivations?” No matter how much data were inputted to that computer, isn’t it still just a big digital library? (Or — should we really be concerned that the Library of Congress, or the internet, will begin thinking on its own?)
I agree that a ‘data-saturated computer [will not] have “intelligence” in any practical sense, much less “understanding” of all that data.’
However, believing that computers are ‘just’ data processing machines is a category mistake (which is why the term IT = Information Technology is unhelpful). Computers are also systems processors, and this is the area where we can anticipate progress towards intelligence in a human sense.
Of course, we don’t know how to achieve this yet, the best we have done so far is to combine knowledge (data) with pattern recognition (systems) to produce machine, as opposed to human, intelligence.
As for ‘understanding’, I agree that we have yet to find a meaningful equivalent to this in a machine context, although Gilbert Ryle’s distinction between ‘Knowing How’ and ‘Knowing That’ (1945) was perhaps a first step.
If a “super intelligent AI” goes from possible to actuality, what would it find as it’s purpose? An endless end game? Would it find itself in a illogical or purposeless comos? If it had true superior intelligence, wouldn’t it play out all possible scenarios and discover existence or it’s own existence, meaningless and thus useless? And proceed to self-destruct, not unlike in some version of a bad Star Trek episode?
Call it insane in the mainframe;)
Matthew,
The question is not why we should be concerned with A.I. but how do we stop human annihilation by the A.I. Terminator? If we can build it, we can use it. After we invented the car, who’s foolish enough to race a car by running on his feet? But putting quantum computer chips in our brain won’t be enough to beat the A.I. Terminator. It’s like trying to put a motorcycle engine in a horse. Eventually we’ll give up and say, why don’t we just get rid of the horse and build a Lamborghini?
The same thing that applies to computers applies to |AI: Garbage in-garbage out. You may program into it all of the knowledge known to man and the calculating ability to do arithmetic at blinding speed, but cannot think outside of the box. A computer cannot play chess unless
it has been programmed to do so. If incorrect information has been programmed into it, it does not have the ability to decide that it is wrong, and any calculation it makes using it is suspect. Even the information that we consider cutting edge today may be proven wrong tomorrow.
Unlike a school child, you cannot tell a computer to forget that information that has been previously taught and ask it to accept new data.
Unfortunately and honestly, I am unable to to understand much of what Dr. Graves has written though I believe I share what I think is his great fear of AI running amuck. I have not come close to staying abreast of the advances in technology in any of its many fields. I do know that strong humanistic properties in terms of the number of people who live by them seem to have advanced very little if at all. Our abilities to cure people of diseases, grow food more efficiently, make the air cleaner, etc. are much improved but are sadly for the most part applied poorly in the real world. We have learned to and do kill people more efficiently. The presence of psychopaths in the “criminal” world” as well as in the financial world have made me think that mental traits as well physical traits are compartmentally separated in our brains to various degrees. The AI scientists must realize that advances in mathematics, chemistry, and the like without improvement in our behavior to each other, may make the world for most of its human inhabitants an even more worse place than it is now.
In the future, our doom sayers about real AI will be viewed like we viewed alchemists. After a 30 year search, not only are we no closer to real AI, we still dont know what would be needed to make it happen. Computers are build of “gate” which do simple arithmetic. Like on plus on equals on. A computer could be made of cogs. Some chemical processing plants still use computers that run off liquid pressure logic circuits. No one has any idea what is needed as the basic unit of intelligence. A computer program controls everything we see on media. Sadly, we wont have a chance to be controlled by our robot overlords. The computers are ALREADY in charge, and they are still stupid.
There is a book entitled, “Where Did I Leave My Glasses?” that explains human memory, and consciousness being related, in nontechnical terms. Given the analogy to a soft writing slate which changes each time memory is tapped, the neural connectome changes all the time. For this reason, the human mind will probably never be duplicated in AI. The Dewey number is in the 100’s, so the book is classified philosophy rather than science. But this explanation made a believer out of me on AI not able to replace a brain.
Forget Asimov, this scenario was foreshadowed in the movie Terminator 2 (1991), in which the super-intelligent AI Skynet adopts its own objectives (not envisioned by its human designer) and launches a nuclear holocaust on August 29, 1997, destroying much of humanity.
The solution? Make sure you have Schwarzenegger available to come back in time and destroy the AI – and the designs that it was based on.
I am unconvinced that non-artificial intelligence exists based on observed human behavior. Our own reasoning abilities have so many flaws (paredolia, compartmentalization, confirmation bias, etc) that there is probably a lot more deterministic responce rather than reasoned response behind much or most of our own actions.
So, if humans are more pre-determined wiring than of free-will, you are saying what’s the difference between a living automaton and a robot? We know there is a big difference: consciousness. Here is an example that humans do have freedom of choice to some extent. There are circumstances we cannot control (natural disasters, etc) but it is how we respond to these that makes us different than a robot.
Interesting article, but let’s not forget another possibility: the intentional design of malevolent AI!
Human beings have scarcely created a tool that didn’t start life as a weapon or was at some point turned into one.
It will be hard enough for us to keep AI from going dangerously beyond our control with the best of intentions let alone the worst.
“It will be hard enough for us to keep AI from going dangerously beyond our control with the best of intentions let alone the worst.”
It will be hard enough for us, with the best of intentions, let alone the worst, to keep AI from going dangerously beyond our control.
Pease’s 23rd Law
Any possibility of ambiguity will annoy or confuse important readers
Dr Grammarfreak
BobDobbs University
Clench CO
Here is a very similar article from several years ago. I recommend it. Find it here–
https://waitbutwhy.com/2015/01/artificial-intelligence-revolution-1.html
The AI Revolution: The Road to Superintelligence
January 22, 2015 By Tim Urban
Matthew,
Wonderfully written, clear-headed analysis. Thank you. Would like to give you attribution while sharing it. Suggest that Twitter links for articles include author’s handle.
Best,
joe
Very interesting but most of the discussion seems to be based on a machine becoming self aware. But consciousness does not appear to be derived from physical laws, and we don’t even know how to pose a question about consciousness. The assumption here is that the brain creates consciousness, but we have no evidence of that, and until a machine is conscious, which may be impossible, we need not worry
hello kevin, this is a subject of personal interest.an ASU Dr.Stuart ?? has written about the location of consciousness lies in the micro-tubules in the brain.a biological end process.these complex patterns could be copied and duplicated by computer.it may not be natural but is pretty dam close.when you factor in human mental disorders,probably far better.too bad we wont be around to see…..
As Comment #8, I’ve found the word I was looking for: palimpsest. Living brain tissue is malleable while computer circuits are not. The memory is like a slate, not a bunch of silicon circuitry. We need not worry about AI replacing human consciousness.
Consciousness is mostly irrelevant to intelligence, and the article does not imply otherwise: this is something you have read into it. Many features of intelligence – insight, for example – occur unconsciously, and consciousness just gets in the way.
As for your statement that we have no evidence that the brain creates consciousness, this is nonsense: we have conclusive evidence. Drugging the brain stops consciousness temporarily, destroying the brain terminates it.
I am quite impressed by this article and I would like to reprint (republish) it in my edited LAW ANIMATED WORLD (http://lawanimatedworld.blogspot.in/). I am a lawyer and social activist. I hope you’ll have no objection for it and request the same.